Continual Learning in Image Classification
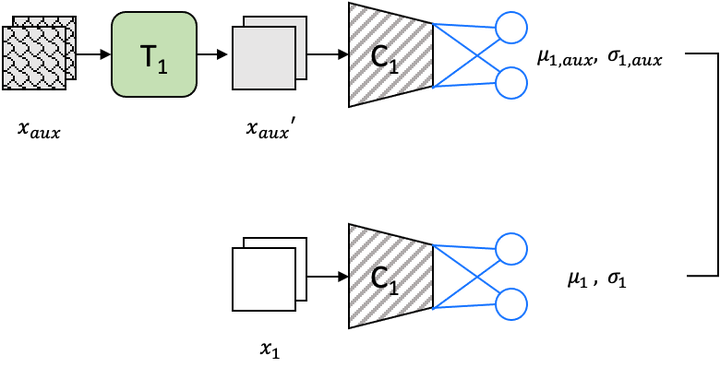
Deep Neural Networks (DNNs) have been successfully applied to many problems in the medical domain. However, the common assumption of having access to the complete training data may not always hold true. Instead, data could be provided by different sources at different points in time. Especially in the medical field, privacy regulations can exacerbate this problem by prohibiting storage of old data. In such scenarios, proper incremental training for DNNs becomes essential to not forget previously learned knowledge. To this end, a recently published method called Deep Model Consolidation (DMC) proposes knowledge distillation with auxiliary data. We use this method as a benchmark and propose improvements on top of it. Furthermore, we present a novel approach to transform auxiliary images to look more like images from old datasets. With experiments on known benchmark datasets as well as a dataset for tissue type classification, we show that DMC and our image transformer-based method can reduce forgetting of previously acquired knowledge.