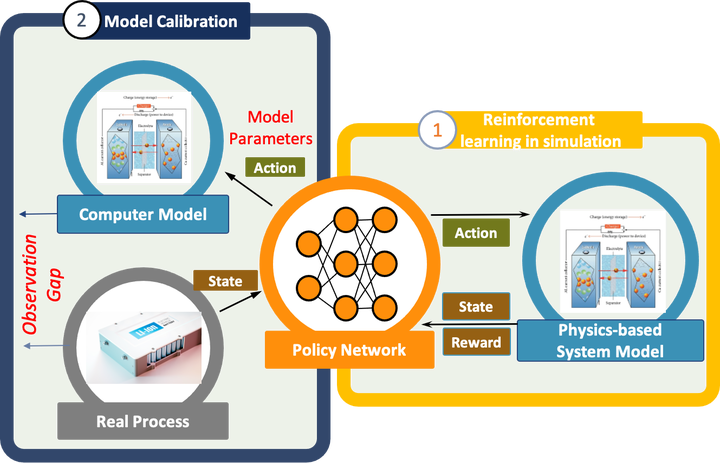
Lithium-Ion (Li-I) batteries are used in many physical assets. To enable a good prediction of the end of discharge of batteries, detailed electrochemical Li-I battery models have been developed. However, these battery models need to be calibrated regularly as the batteries degrade. In this paper, we implement a model-free Reinforcement Learning-based framework for reliably and efficiently inferring calibration parameters of battery models. The framework enables real-time inference of the battery degradation parameters in order to compensate the reality-gap from the observations. Most importantly, the proposed methodology does not need any labeled data samples, (samples of observations and the ground truth calibration parameters). Furthermore, to increase the stability of the parameter inference, we incorporate lyapunov energy decreasing condition as a regularizer for our policy network objective. Lyapunov regularizer enables that the inference tracking of the parameters converges to an optimal, no matter the initial condition. The experiments show impressive results compared to Kalman Filter-based approaches. Moreover, this framework is highly general and can be employed in calibration of other computational models.